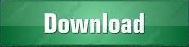
- Visualize decision tree python without graphviz install#
- Visualize decision tree python without graphviz zip#
plot ( X_test, y_1, color = "cornflowerblue", label = "max_depth=2", linewidth = 2 ) plt. scatter ( X, y, s = 20, edgecolor = "black", c = "darkorange", label = "data" ) plt.
predict ( X_test ) # Plot the results plt. fit ( X, y ) regr_2 = DTR ( max_depth = 5 ) regr_2. rand ( 16 )) # Fit regression model regr_1 = DTR ( max_depth = 2 ) regr_1. ravel () print ( "shapes: \t X: \t ", X. Import numpy as np from ee import DecisionTreeRegressor as DTR import matplotlib.pyplot as plt # Create a noisy sinusoidal dataset rng = np. target ) plot_tree ( clf, filled = True ) plt. suptitle ( "Decision surface: paired features" ) plt. RdYlBu, edgecolor = 'black', s = 15 ) plt. scatter ( X, X, c = color, label = iris.
Visualize decision tree python without graphviz zip#
feature_names ]) # Plot the training points for i, color in zip ( range ( n_classes ), plot_colors ): idx = np. arange ( y_min, y_max, plot_step )) plt. arange ( x_min, x_max, plot_step ), np. subplot ( 2, 3, pairidx + 1 ) # decision boundry x_min, x_max = X. Prebalancing datasets prior to fitting will help.įor pairidx, pair in enumerate (,, ,, , ]): X = iris. Prone to bias in unbalanced dataset problems.Some concepts, such as XOR, do not lend themselves well to tree techniques.Cannot guarantee globally optimal solutions due to algorithms choosing locally optimal answers at each node.Prone to instability due to data variations causing completely different trees to be built.Pruning, fixing maximum leaf sizes and fixing maximum tree depths help.
Prone to overfitting with overly complex trees. Can be validated with statistical tests. Can handle numerical & categorical data (note: Scikit-Learn implementation does not support categories at this time.). Computational cost is logarithmic, ie log(#datapoints). Visualize decision tree python without graphviz install#
Import via conda install python-graphviz.
Export results to Graphviz format using export_graphviz. Simple to understand, interpret & visualize using plot_tree. Non-parametric technique for classification & regression. This article goes deeper into explaining how the algorithm typically makes a decision. The above excerpt has been taken from an article I wrote on understanding decision trees. The algorithm tries to split the data into subsets so that each subgroup is as pure or homogeneous as possible. This approach is technically called recursive partitioning. The algorithm of the decision tree models works by repeatedly partitioning the data into multiple sub-spaces so that the outcomes in each final sub-space are as homogeneous as possible. Branches: arrows connecting nodes, showing the flow from question to answer. Terminal Nodes/Leaf node: Nodes that predict the outcome. Root Node: The node that performs the first split. It can be used both for regression as well as classification tasks. A Decision Tree is a supervised learning predictive model that uses a set of binary rules to calculate a target value. It can be installed with pip install dtreeviz butrequires graphviz to be pre-installed.īefore visualizing a decision tree, it is also essential to understand how it works. For the installation instructions, please refer to the official Github page. We’ll see how the dtreeviz scores over the other visualization libraries through some common examples in the following sections. These operations are critical to for understanding how classification or regression decision trees work. With dtreeviz, you can visualize how the feature space is split up at decision nodes, how the training samples get distributed in leaf nodes, how the tree makes predictions for a specific observation and more. The visualizations are inspired by an educational animation by R2D3 A visual introduction to machine learning. The dtreeviz library plugs in these loopholes to offer a clear and more comprehensible picture. The size of every decision node is the same regardless of the number of samples. The visualization returns the count of the samples, and it isn't easy to visualize the distributions. There are no legends for the target class. It is not immediately clear as to what the different colors represent. There are some apparent issues with the default scikit learn visualization, for instance: Visual comparison of the visualization generated from default scikit-learn(Left) and that from dtreeviz(Right) on the famous wine quality dataset | Image by AuthorĪs is evident from the pictures above, the figure on the right delivers far more information than its counterpart on the left.